Behaviour analysis in complex environments
Abstract
Complex environments pose significant challenges for understanding behaviour. Humans exhibit intricate behaviours in complex environments, especially during chaotic events where interactions between individuals and their surroundings become highly dynamic and unpredictable. Similarly, animal behaviour in natural settings exhibits complex patterns, with diverse species exhibiting unique behaviours, making analysis and interpretation difficult. Understanding and interpreting these behaviours is essential for applications like crowd management, emergency response, public safety and wildlife management.
To address these challenges, we first present Chaotic World, a large-scale multi-modal dataset with fine-grained annotations of human actions, interactions, and sounds in chaotic situations. This dataset facilitates the development of advanced models for spatiotemporal action localization, behaviour graph analysis, spatiotemporal event grounding, and event sound source localization in chaotic situations. We further propose a unified multi-task learning model that jointly trains four key tasks related to chaotic event analysis.
A dynamic knowledge pathfinder optimizes the learning process by automatically selecting task-relevant and shared features, enhancing the model’s ability to generalize across tasks. The results show that the IntelliCare model demonstrate good performance in chaotic event analysis with 16.62 PredC1s in behaviour graph analysis, 47.38 m-tIoU in spatiotemporal event grounding, 57.37 cIoU in event sound source localization and 16.25 frame-Map in spatiotemporal action localization.
Lastly, we propose a collaborative action recognition model designed to recognize animal’s actions across various species, including unseen ones. Leveraging meta-learning, the model effectively generalizes by learning from both general and species-specific action features. This approach demonstrates strong performance in recognizing actions of previously unseen animal types, extending the applicability of action recognition models. The results demonstrate the CARe model’s effectiveness, achieving an accuracy of 39.7% in action recognition of unseen animals. Ablation studies demonstrate the significance of specific features, general features, and spatially aware weighting, which collectively enhance performance.
Speaker’s profile
Ng Xun Long is a PhD candidate at Singapore University of Technology and Design (SUTD). He received first class honours for his B.Eng. (Hons) from University of Glasgow, in 2020. His Ph.D. research focuses on computer vision, behaviour analysis and multimodal models.
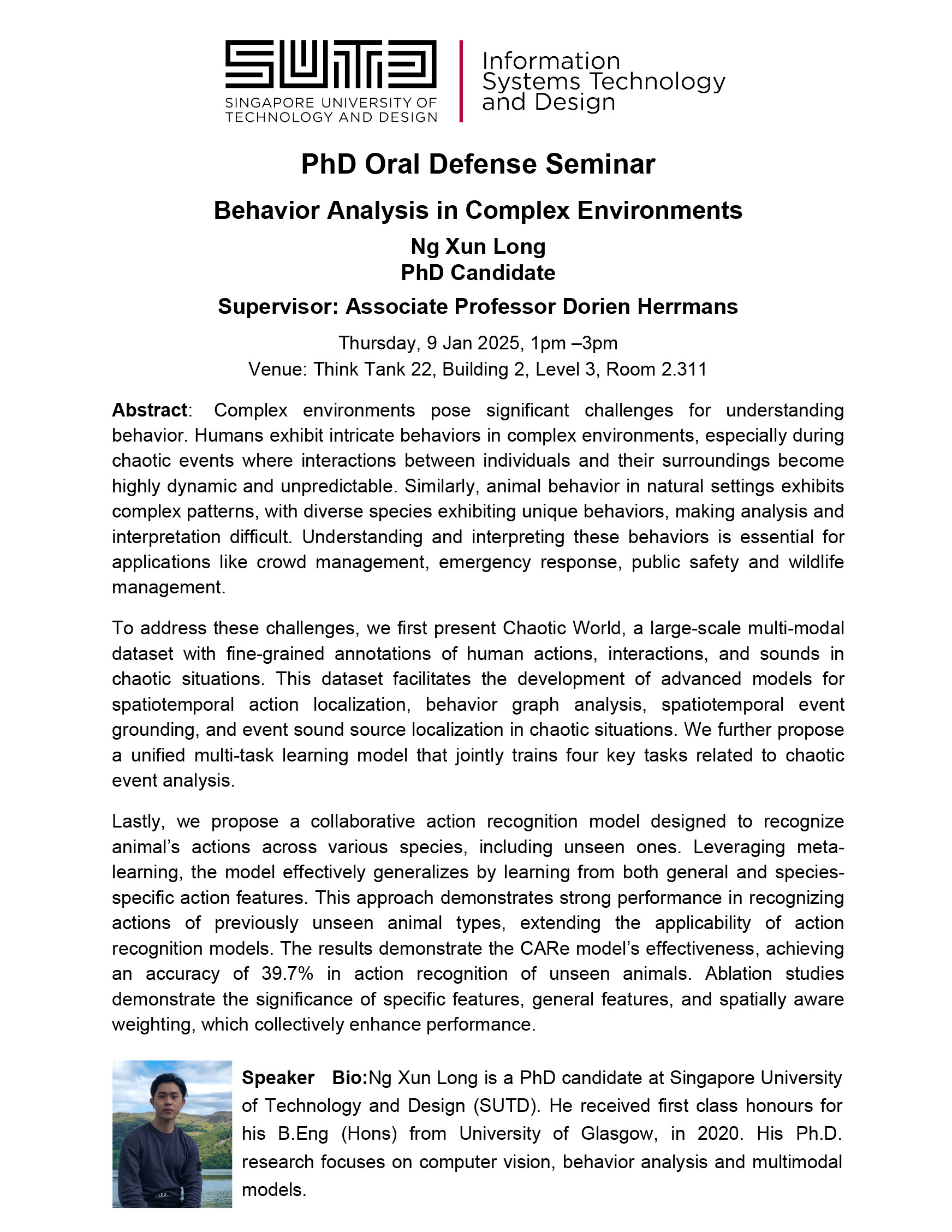