Guided cooperation for multi-agent teams
Abstract
Cooperation is defined as teamwork where the behaviour of individual agents is aligned towards a common purpose, and agents can leverage teammates’ help to improve overall task performance. We argue that without this shared direction, agents are not truly cooperating. To cooperate, a team of agents requires guidance to provide direction as well as ensure that this direction is shared among the agents, whether it is provided by a central authority or developed through consensus arising from intergroup discussion. Centralised guidance allows agents to augment their behaviour with knowledge beyond their limited perspective, align their actions towards a shared purpose, and even synchronise these cooperative efforts with teammates for precise joint manoeuvres. This allows the team of agents to tackle tasks that would be impossible for a single agent acting alone. Guidance can originate from a central authority or arise from inter-agent communication for the team to lead itself. We study various degrees of centralisation in cooperation mechanisms, spanning from fully centralised planning-based approaches to fully decentralised communicating agents. In the centralised setting, we address the problem of multi-target search using multiple searchers in a dynamic environment. We propose a theoretical guarantee of multi-target capture using a cluster-based search. Our centralised approach was tested through simulation using real-world wind and current data while accounting for the physical property of the target object based on the OpenDrift framework. This is one of the first works to provide theoretical guarantees for multi-target capture in a dynamic environment. For the partially decentralised setting, our research in communication learning and strategy learning culminated in an analysis of how to fine-tune priors and sequence primitives within the context of multi-agent strategy learning. Our examination of inter-agent influence among implicitly cooperating agents gave us insights into how agents affect one another while attempting to synchronise their cooperative behaviour. Through that study of social behaviours for task-agnostic cooperation, we discovered a novel possibility of how mixed teams of high-performing agents can be trained to work together without the traditional training signal of task rewards. Instead, agents within the team can teach each other to cooperate, allowing for unsupervised adaptation of agents to a new team without the use of task reward. We demonstrate our work in multi-agent reinforcement learning (MARL) on the Starcraft Multi-Agent Challenge (SMAC) and Multi-Agent MuJoCo (MaMuJoCo) benchmarks.
Speaker’s profile
Pamela is a PhD candidate at the Information Systems Technology and Design (ISTD) pillar of the Singapore University of Technology and Design. She received Honours for her B.Eng. (Hons) from the Singapore University of Technology and Design in 2019, specifically following the Artificial Intelligence Track. Her research explores various forms of guided cooperation that enable cooperative behaviours in multi-agent teams.
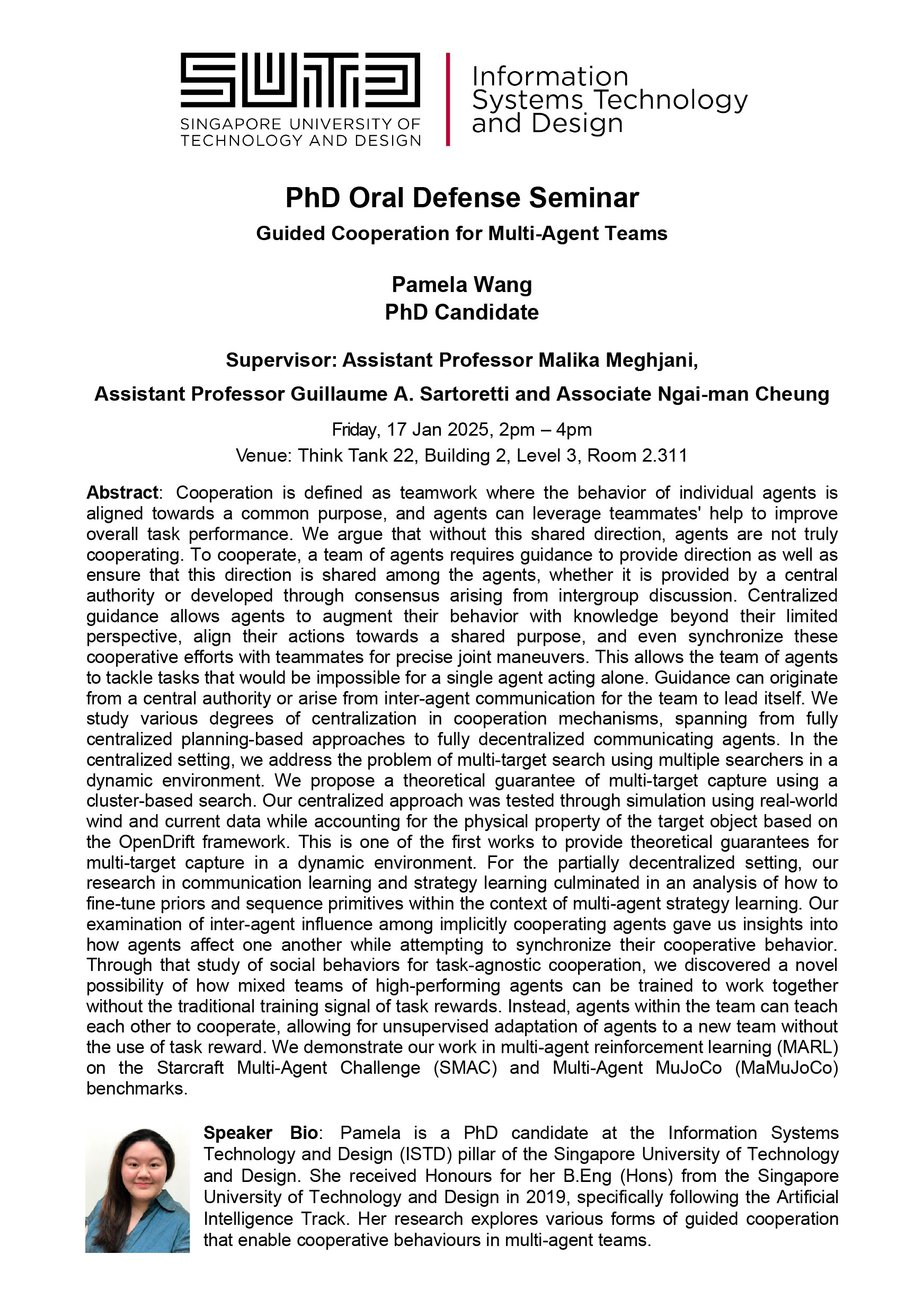