Learning text styles: a study on transfer, attribution, and verification
Abstract
This thesis advances the computational understanding and manipulation of text styles through three interconnected pillars: (1) Text Style Transfer (TST), which alters stylistic properties (e.g., sentiment, formality) while preserving content; (2)Authorship Attribution (AA), identifying the author of a text via stylistic fingerprints; and (3) Authorship Verification (AV), determining whether two texts share the same authorship. We address critical challenges in these areas by leveraging parameter-efficient adaptation of large language models (LLMs), contrastive disentanglement of stylistic features, and instruction-based fine-tuning for explainable verification.
First, for TST, we conduct a comprehensive survey and reproducibility study of 19 state-of-the-art algorithms, establishing benchmarks across diverse datasets. Building on these insights, we introduce LLM-Adapters, a unified framework for parameter-efficient fine-tuning (PEFT) that enables cost-effective adaptation of LLMs for style-centric tasks. This culminates in Adapter-TST, a novel architecture that models multiple stylistic attributes (e.g., sentiment, tense) using lightweight neural adapters. Adapter-TST achieves superior performance in multi-attribute transfer and compositional editing while reducing computational costs by 80\% compared to full fine-tuning.
For AA, we propose ContrastDistAA, a contrastive learning framework that disentangles content and style features to address performance degradation under topic shifts. Our method advances both individual-level attribution and regional linguistic analysis, achieving state-of-the-art accuracy by isolating culturally influenced stylistic patterns.
In AV, we bridge the gap between LLM capabilities and forensic analysis with InstructAV, an instruction-tuned model that jointly optimizes verification accuracy and explainability. By aligning classification decisions with human-interpretable rationales, InstructAV outperforms ChatGPT and specialized baselines across multiple datasets, demonstrating the viability of LLMs for forensically rigorous tasks.
Collectively, this work establishes a paradigm for efficient, interpretable, and multi-faceted text style analysis. Our contributions span novel architectures (Adapter-TST, ContrastDistAA, InstructAV), reproducible benchmarks, and open-source tools (LLM-Adapters), enabling future research in style- aware NLP applications ranging from personalized text generation to forensic linguistics.
Speaker’s profile
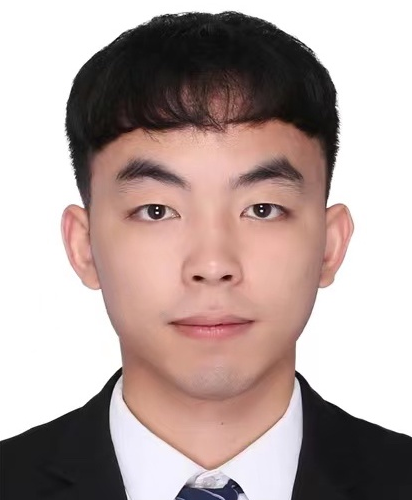
I am a final-year PhD student at the Singapore University of Technology and Design (SUTD), where I am fortunate to be advised by Professor Roy Ka-Wei Lee. Before that, I got my master’s degree on Computer Science at the UESTC, where I was supervised by Professor Dai Bo. My undergraduate studies were also at UESTC, where I earned my bachelor’s degree on Applied Mathematics from the school of Mathematical Sciences. My research interests encompass various domains, including Large Language Models, Multi-modal LLMs, Math Word Problem Solving, and Text Style Transfer.