SUTD develops intelligent model simulator that maps complex phenomena of memristor memory
SUTD – Qishen Wang, Karthekeyan Periasamy, Natasa Bajalovic and Desmond K. Loke
Chang Gung University – Qishen Wang, Karthekeyan Periasamy, Yi Fu, Ya-Ting Chan, Cher Ming Tan and Jer-Chyi Wang
Ming Chi University of Technology – Cher Ming Tan and Jer-Chyi Wang
Chang Gung Memorial Hospital – Jer-Chyi Wang
Memristor memory technology is one of the most promising candidates for next generation edge computing. SUTD led in the development of an intelligent model simulator that predicts complex phenomena of memristor memory to enable the production of a cheaper faster memory.
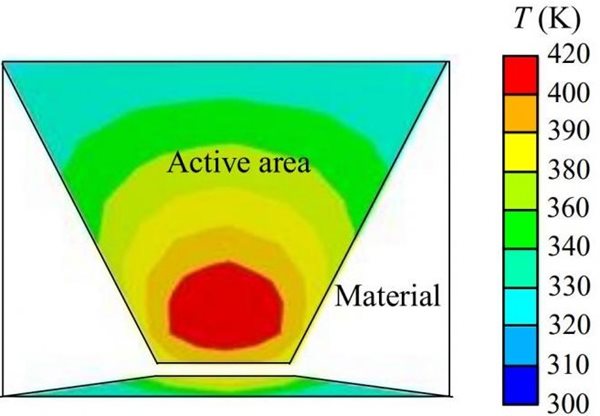
Snapshot depicts the temperature profile of the memristor memory model.
Memristor memory technology is set to revolutionise computers the world over as it is touted as one of the most promising candidates used for next-generation edge computing. This technology is attracting a lot of attention for replacing flash due to its implementations in high-efficient in-memory computing, machine learning and neuromorphic computation. Realising a model to predict the phenomena of memristor memory technology accurately is essential as this will allow engineers to design systems with more efficient behaviors for making a cheaper, faster memory.
Currently, a wide range of experimental and modelling studies have been reported to understand the transport process, which occurs when a current passes through the device. Several important characteristics, such as applied voltage, electric field, material constants and so on, are applied in the model simulators for predicting this process. Transport process can be analysed by various models with the help of simulation tools and advanced observation technologies.
A Singapore-led collaboration has successfully created a simulator using both electronic and thermal components to make a “transport pattern”. The team then used this hybrid platform to map a long-standing challenge in memory technology: the transport process under many conditions.
Corresponding author, Assistant Professor Desmond Loke from SUTD, said, “What we have done is take two different components of a model, memristor model, which show different behaviour from each other. When these are put them together, one can create a transport pattern that is up to 700 times more accurate than traditional models”.
Due to Joule heating, the potential temperature of the device increases and yield variations in electronic properties, such as mobility of electrons and depth of traps. These variations influence the analysis and prediction of transport behaviour of memristor memory.
By considering the models of transport behaviours and assumptions related to the electron mobility and trap depth, the transport behaviours of memristor memory cells can be precisely predicted. Furthermore, the rich transport and switching behaviours can be fully accounted for by describing device characteristics obtained by an entirely new set of general current-limiting parameters.
This research was published in the journal AIP Advances in August. The lead author is Mr Qishen Wang and the project is led by Dr Natasa Bajalovic and Dr Desmond Loke from the Singapore University of Technology and Design (SUTD) and Dr Jer-Chyi Wang from Chang Gung University. It is supported by the Ministry of Education, Singapore, Singapore University of Technology and Design and Chang Gung university.
Reference:
Analytical Modeling Electrical Conduction in Resistive-Switching Memory through Current-Limiting-Friendly Combination Frameworks, AIP Advances, (8), (DOI: 10.1063/5.0019266)
Acknowledgements:
The authors thank Professor Jia-Hong Sun of Chang Gung University for model discussions and software support. This work was supported by the Ministry of Science and Technology, R.O.C. (Contract No. of MOST 108-2218-E-182-003 and 108-2221-E-182-027), and Chang Gung Memorial Hospital, Linkou, Taiwan (Contract Nos. CMRPD2H0132, CMRPD2J0051, and BMRPA74). The authors acknowledge financial support from the Ministry of Education, Singapore (Grant Nos. MOE 2017-T2-2-064 and SUTDT12017003); SUTD-MIT International Design Center, Singapore; and the SUTD-ZJU IDEA Grant Program [No. SUTD-ZJU (VP) 201903]. Q. Wang thanks the Singapore University of Technology and Design for scholarship support.